Best Practices: Generating Multiple Expression Constructs
Proteos is an industry leader in the production of high-quality recombinant proteins for drug discovery research. There are many variables to consider when producing novel recombinant proteins, and for those with no prior precedent for recombinant protein production, it may be beneficial to generate multiple variants of a protein construct.
Adding an affinity tag to a target protein can simplify purification, detection, and downstream assays. Moreover, affinity tags that also act as fusion partners can enhance the solubility and stability of recombinant proteins. However, the size, number, and placement of the affinity tags can alter the properties of the protein. Therefore, it is important to generate multiple expression constructs and test their efficacy in pilot experiments. For example, a large tag or multiple small tags can change the protein’s structural conformation. If this is the case, a smaller tag or fewer tags may be a better alternative. The placement of the tag can also affect the function and stability of the protein. Therefore, it is helpful to determine empirically if the tag should be placed at the N-terminus or C-terminus of the protein. Finally, protease removal of the tag(s) may be desired after expression, depending on the subsequent purification steps and downstream applications. In this instance, a protease recognition sequence needs to be included during construct design. Tag removal, especially for fusion partners, should be tested in pilot experiments to ensure that it does not render the protein insoluble.
At Proteos, every project is customized to the customer’s specific target, need, and end-use. We will design a milestone driven workflow that can include generation of multiple constructs, if deemed necessary for your project. This allows our highly-skilled scientist to make data driven decisions which mitigate risk and increase the likelihood of successful project outcomes.
Our next Best Practices blog will focus on performing small-scale expression optimization.
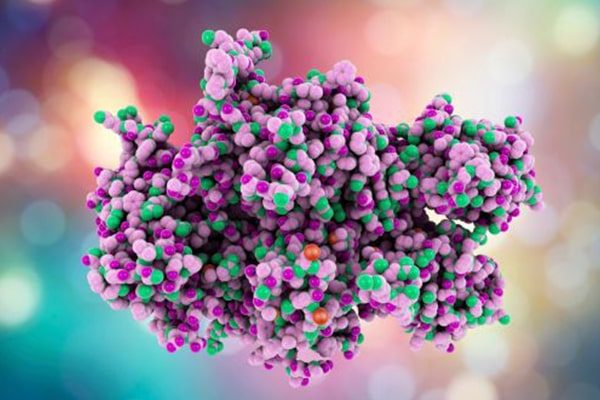